Utars 1.5 for Better GUI Tasks with Vision-Language Agents
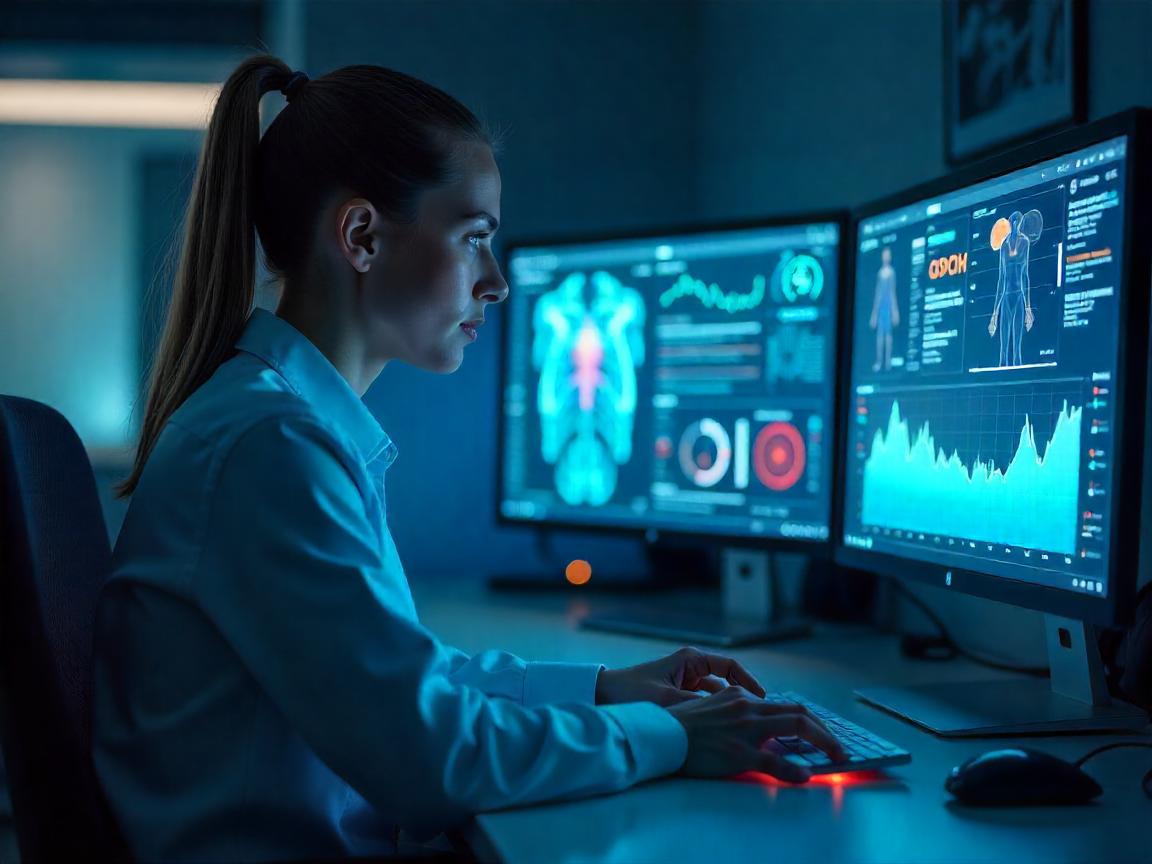
The development aim is to create computational hardware that detects screen content for humanlike interactions. The newest innovation from Bite Dance known as Utars 1.5 brings forth this capability. Utars 1.5 presents itself as more than a simple tool because it introduces AI-powered screen control applications that process computer displays as extensive images. This innovation has the power to revolutionize automated work and software testing along with gaming functionalities. We should examine the workings of Utars 1.5 together with its exceptional features.
What Is Utars 1.5? An Overview of the Next-Gen Vision-Language Agent
Utars 1.5 represents an intelligent AI system which operates computer mice, touch screens and keyboards independently. The system operates without script or coding patterns because it views entire displays as one consolidated image. The system examines the displayed content to comprehend the arrangement and subsequently imitates human screen interaction. Normal automation tools need complex codes and detailed instructions whereas Utars follows a screen-based “map” approach. The system directly examines the screen while it autonomously performs operations and progress through tasks which results in quick adaptable functionality.
The advanced graphical interface of Utars enables it to interpret and comprehend elements displayed on screens.
Visual Element Recognition and Synthesis
Utars possesses vision capabilities to view everything shown on screens. The tool successfully locates and identifies boxes alongside icons and labels and detects colors and small hover states. Through its system utars will show you the precise spot of the save button while pointing out menu icons. Through comprehensive combination of descriptions such as “a blue square with a floppy disk icon” Utars offers better comprehension abilities. Complex interfaces and UI changes become simpler to navigate for Utars because of its ability to detect everything shown on the screen.
Multi-Modal Perception Data and Grounding
The sensor constructs multiple kinds of perception data to validate its observations. The system produces distinct forms of understanding from which element descriptions and layout captions serve as two examples. The system indicates screen regions through colored markers that allow its language to match particular parts of the display. Such methods enable the system to hit specific buttons or icons when the screen becomes disorganized or when UI elements transform unexpectedly.
Native Action and Control: How Utars Executes Tasks Like a Human
Built-in Action Space and Primitives
The basic set of commands are built into the Utars systems. Utars possesses capabilities for clicking along with dragging and scrolling while it also types and waits for specified periods. The system provides mobile users with back navigation and extended button presses as possible commands. The system detects desktop hotkeys and right-click functions. As a secondary functionality the system provides actions to complete workflows and request human intervention when operations become stuck. Various combined actions enable the AI to function as a person operating the monitor.
Learning from Human Traces and Multi-Step Tasks
During training Utars learned from millions of real procedures that consisted of approximately 15 individual steps. Human behavior traces demonstrate the steps people follow in using different digital activities which involve browser opening along with form filling and menu navigation. Utars acquires the ability to execute complete user processes by understanding complete workflows instead of isolated touches. The system has the ability to manage Windows applications alongside Android devices as well as web pages at a professional level.
The process of reasoning and decision-making functions through two thinking systems known as System One and System Two.
Fast, Intuitive Reasoning (System One)
Simple tasks require two basic instructions to complete either a click or entering text. Utars perform rapid decision-making through instant decision patterns. The system detects buttons while understanding all states before performing its tasks with minimal delay. The basic automation process operates efficiently due to this system.
Deliberate, Chain-of-Thought Reasoning (System Two)
Tasks requiring an advanced approach must receive proper planning stimuli. The design process of Utars includes problem simplification through stepwise approaches that resemble human thinking methods. Through this style of thinking the system evaluates possible solutions while recognizing specific stages which it adjusts if a step does not progress correctly. Users find success with their chain-of-thought approach while performing lengthy forms and problem troubleshooting tasks.
Improving Accuracy with Thought Sampling
The decision-making process at Utars consists of testing multiple logical approaches. The system develops multiple logical solutions after which it chooses the most suitable one. The “best of N” analysis technique enhances achievement rates particularly during complex circumstances. Each possible approach is evaluated until the shortest one is selected.
The system makes use of error learning to reinforce its knowledge of optimal best practices
Capturing and Annotating Failures
Utars occasionally commits mistakes during its operations. After humans review these system errors they identify both the causes of failure and the necessary solutions. The AI system receives feedback through this loop that leads to cumulative improvements in its performance level.
Preference Optimization and Reinforcement
DPO functions as a process which motivates successful choices yet punishes wrong choices. The training process becomes effective at increasing successful outcomes. The success rate of Utars increased substantially after focusing on special desktop benchmarks where it shifted from achieving 17% to surpassing 24%.
Benchmark Performance and Real-World Applications
Desktop and Mobile Benchmarks
Modern tests reflecting professional conditions display that Utars 1.5 achieves superior performance compared to numerous contemporary AI agents. The Windows desktop success rate for Utars reaches 42% success rate which surpasses GPT-4-based systems. The 7 billion-parameter model on Android achieves 64% task success which outperforms previous versions of this model. Specific tools assist the mobile UI task performance of Utars 1.5 to reach nearly 94% accuracy.
Gaming and Complex Tasks
The capability of Utars extends to solving games which include Infinity Loop and Snake Solver. The new gaming capabilities of Utars 1.5 surpass older models which succeeded in just 1% of Minecraft mining tasks but now achieve 42% success rate across 200 mining tasks. The system shows its best performance during web navigation because it finishes more than 84% of web browsing tasks.
Scale and Model Variants
Bigger isn’t always better. Specifically trained smaller models prove better at task completion than general and large models. A desktop-oriented model with 7 billion parameters excelled above competitors even though these competitors had greater parameters targeted at different application domains.
Open-Source Deployment and Customization Opportunities
Utars team released its weights through Hugging Face under the terms of Apache 2.0 license. Through the Apache 2.0 license users can apply custom modifications to the models without needing expensive subscription services. The repositories receive scripts for training and action schemas along with screen-capture automation tools that enable developers to design their proprietary AI-driven automation tools.
Evolution of GUI Automation: From Rule-Based to End-to-End Neural Models
The previous method of automation operated with rule-based systems which implemented fixed scripts. The previous systems became unstable when user interfaces underwent any modification. After rule-based systems a new generation of language models entered the market featuring AutoGPT as their representative. These systems prove inadequate as they need frequent maintenance to operate correctly. The AI system Utars operates through data-trained vision-driven operations. The system adapts rapidly through new data training following the analysis of real data.
Core Capabilities Driving Utars 1.5
For its operations, Utars requires four essential abilities:
- Preception involves the ability to recognize screen contents.
- The successful completion of mouse movement with precise screen interaction counts as an action.
- Reasoning: Planning and problem-solving.
- Memory: Remembering recent steps and long-term lessons.
Their model successively undergoes three training phases including basic pretraining but also fine-tuning with high-quality data and finally reinforcement learning assisted by human feedback. Each stage of the training procedure makes the AI system more effective for performing real-world operations.
Future Directions and Broader Impacts
The AI system features wider applications than computer screen control functions. The model features versatility to adapt to different fields including medical diagnostics as well as gaming systems. Developers maintain control of the core engine structure because OpenNMT-tf features a flexible design enabling new UI type integration and custom data source adoption. Artificial intelligence agents will someday manage sophisticated workflow tasks across various sectors while eliminating human-handled operations to boost operational performance.
Conclusion
The new version Utars 1.5 provides significant improvements to general user interface automation capabilities. A universal smart agent which functions identical to human beings through sight thinking and active movements. Through its fast operation and high reliability and customizable features this system presents an advanced approach to automated work which previously required complex programming codes. The routine task takeover by AI begins through Utars which enables applications management together with website navigation and game operation. This marks the beginning point for the future of open movement-based artificial intelligence that operates the mouse.