DeepSeek V3: The Open-Source AI Revolutionizing Efficiency and Accessibility
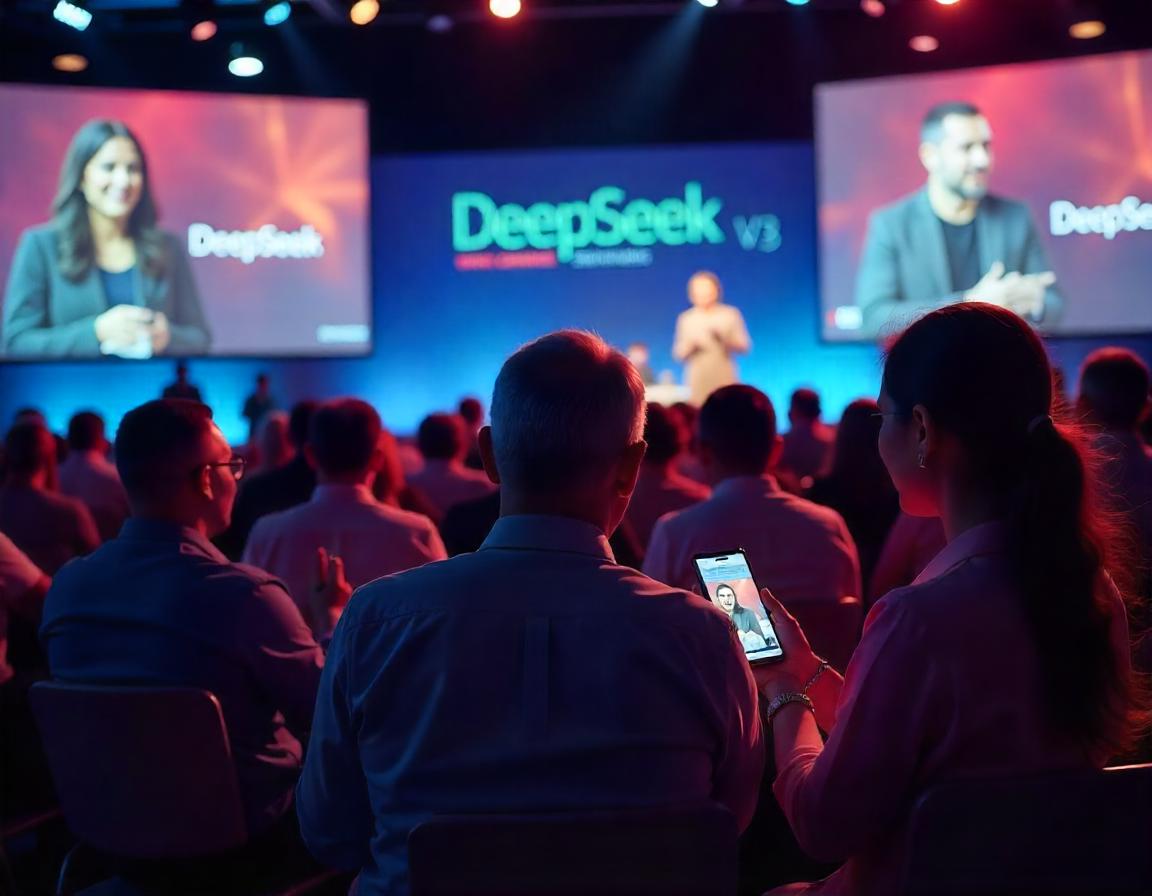
The current version of DeepSeek, DeepSeek V3, is much more highly designed and promising than any other AI system. This deep learning tool, DeepSeek AI, unveiled in December 2019, has an open-source benchmark character that has altered the scenario of large language models; it has 671 billion parameters. They improve speed and effectiveness by activating only 37 billion parameters per token processed, which is their outstanding concept. The versatility of this compound clearly suggests application in major areas of industry and research in many fields.
DeepSeek V3’s Architecture: A Blend of Innovation
The architecture of DeepSeek V3 stands out due to its combination of two key frameworks: ME with Multi-head Latent Attention (MLA).
- Mixture of Experts Framework: This enables the model to choose which sections to activate based on the task at hand, enhancing effectiveness.
- Multi-head Latent Attention (MLA): This mechanism ensures the model focuses on the most relevant data, avoiding distractions from unnecessary information.
For instance, when DeepSeek V3 solves a math problem, it calls on sub-networks with mathematical problem-solving aptitude. In the case of a coding problem, it goes to the programmers with an understanding of languages and algorithms. Such flexibility enables DeepSeek V3 to transition to different workloads easily and not necessarily remain exclusive to a specific task such as analysis, code check, or discussion of philosophical concepts.
The Training Process: Efficiency and Cost-Effectiveness
DeepSeek V3’s training process is both extensive and cost-efficient.
- Extensive Dataset: The model takes in approximately 14.8 trillion tokens, roughly equivalent to 11.1 trillion words. This will guarantee extensive coverage of several areas of interest, including science, technology, literature, and mathematics.
- Cost-Effective Innovations: Additionally, saving the dual pipe algorithm and the mixed-precision training (8-bit format) is concerned with reducing the total expenses incurred during the training phase. The overall cost came to approximately 5.576 million with 2788 million GPU hours employed, significantly less than many similar models employ.
These innovations enhance efficient utilisation of the available hardware to meet the intended objectives without necessarily requiring large capital investment so that organisations of all sizes can apply the model to various tasks.
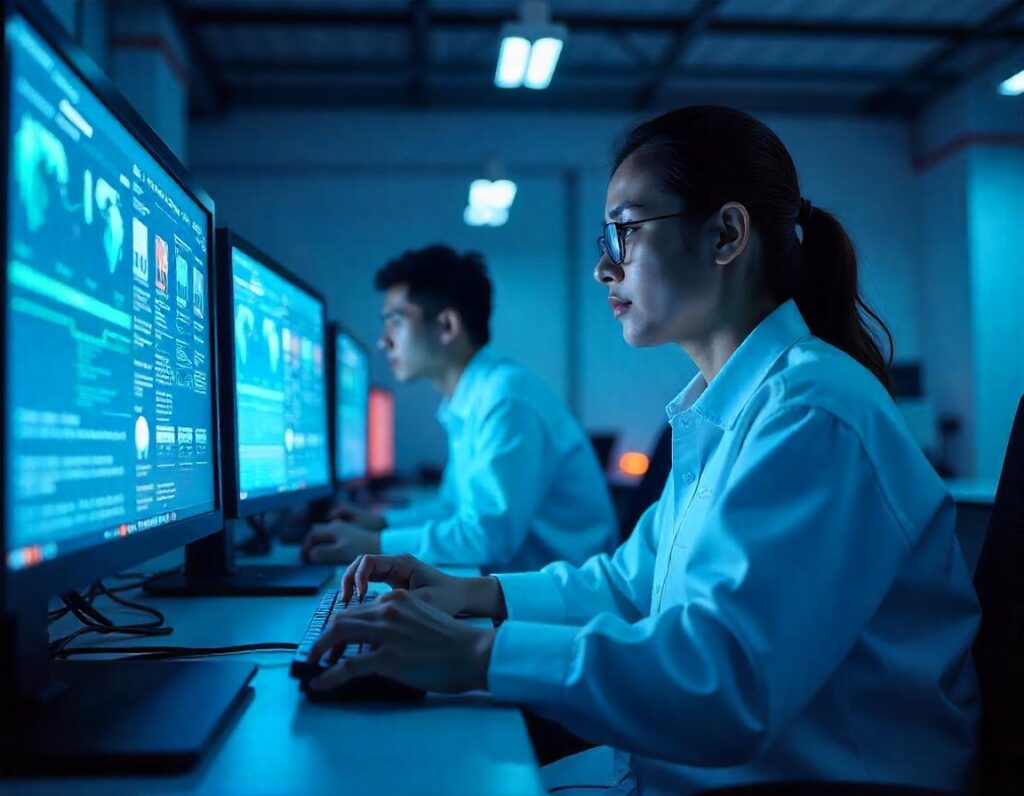
DeepSeek V3’s Performance and Benchmarks
DeepSeek V3 has delivered impressive performance across several benchmarks:
- Math500: Achieved a score of 90.2, showcasing strong mathematical reasoning.
- MML and MML Pro: Scored 88.5 and 75.9 respectively, demonstrating capability in high school and college-level subjects.
- Live Codebench and Codeforces: Successfully generates working solutions for programming tasks.
These capabilities prove that DeepSeek V3 can perform relevant tasks such as software development and data analysis while demonstrating high-performance competitiveness compared with other language models.
Open Source Accessibility and Community Collaboration
One of the significant improvements with the DeepSeek V3 release is that the model shall be open-source. This is thanks to libraries like GitHub and Hugging Face, which allow anyone to give it a try and contribute. This openness fosters community collaboration, leading to:
- Contributions from Developers: Third-party developers have already begun fine-tuning the model, optimizing it for local standards.
- New Features and Improvements: The community can quickly identify issues or suggest enhancements, ensuring the model continues to evolve.
Thus, the proposed approach is conducive to innovation and can identify weaknesses, improving the model’s total reliability.
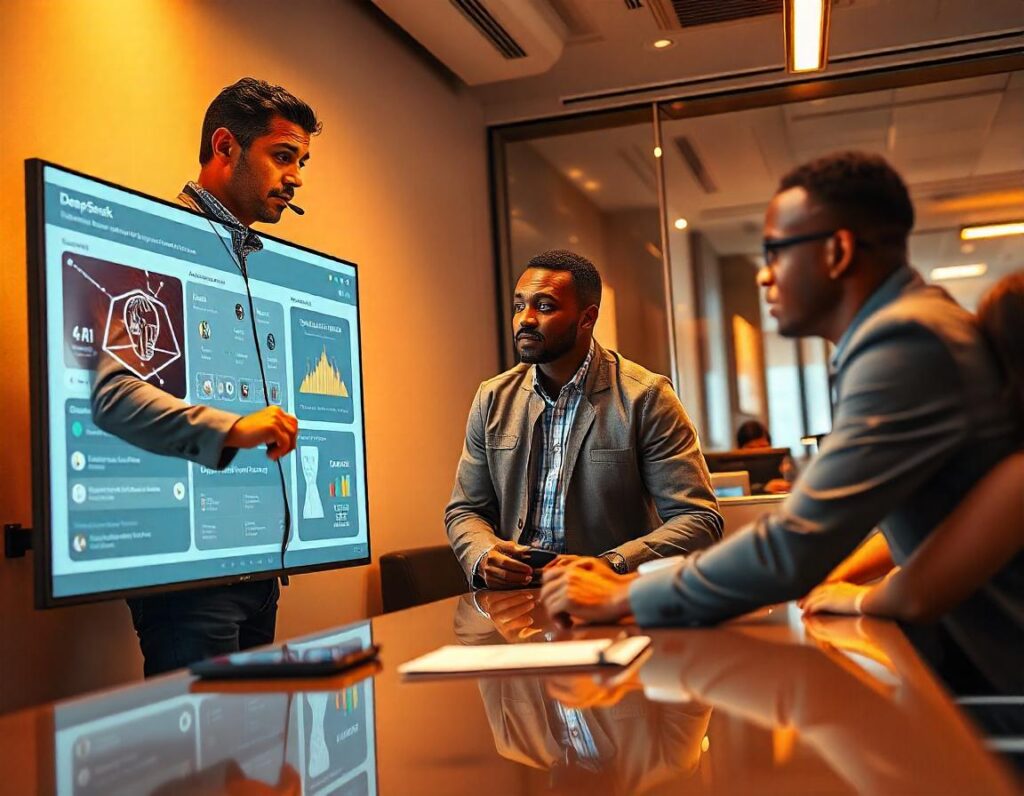
The Future of AI: DeepSeek V3’s Impact and Implications
The quantitative hedge fund Highflyer Capital Management has been crucial for providing infrastructure to DeepSeek V3. Its funding supplied computation during the main training phases; yet again, it’s a nice mix of funding and an open-source ethos.
As organizations adopt DeepSeek V3, its influence spreads across:
- Education: Teachers use it to create personalized tutoring experiences that adapt to individual student needs.
- Business: Customer service departments are testing its ability to respond to inquiries, sometimes generating empathetic responses that can calm frustrated customers.
- Research: Data analysts utilize its advanced reasoning for exploring vast datasets, identifying trends more efficiently than human teams.
Establishing a new benchmark for other aspiring AI projects, DeepSeek V3 proves that one does not have to invest billions of dollars for its development. This efficient model might encourage other labs to aim to strengthen and improve the existing spare parts and algorithms.
Conclusion: A New Era of Accessible and Efficient AI
This means that DeepSeek V3 is not just a very large language model but an example of a completely different approach to AI’s ability to offer working solutions in many areas. As such, emphasizing efficiency, accessibility, and community involvement threatened traditional closed systems. Next, with technology development in the future, ideas such as DeepSeek V3 demonstrate that everyone with a prototype can find the tools to create new ideas for subsequent generations.